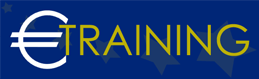

Predictive Modeling and Machine Learning
Overview:
Introduction:
The Predictive Modeling and Machine Learning training program teaches practical application of predictive modeling and machine learning techniques for data analysis. Participants learn to build and deploy models, interpret results, and drive data-driven insights across industries.
Program Objectives:
At the end of this program the participants will be able to:
-
Learn what machine learning really means.
-
Understand the main distinctions between machine learning and data analysis.
-
Integrate testing and sample validation into machine learning models
-
Please provide a summary of the top analytical solutions.
-
Apply precise estimation using comprehensive prediction models.
Targeted Audience:
-
Data scientists and analysts seeking to enhance their skills in predictive modeling and machine learning.
-
Business professionals interested in leveraging data-driven insights for decision-making.
-
Software engineers looking to implement machine learning algorithms into their applications.
-
Researchers and academics exploring the applications of predictive modeling and machine learning in various fields.
-
Anyone interested in advancing their understanding of data science and machine learning techniques for practical use.
Program Outline:
Unit1:
Analysis of the Data and Simple Regression:
-
Means and proportions of two groups are examined.
-
Using a single chart to profile two groups.
-
Comparing the averages and proportions of several groupings.
-
Combining numerous group profiles into a single chart.
-
Regression versus correlation.
-
Sensitivity evaluation of numerical variables.
Unit2:
Logistic and Multiple Regressions
-
Machine Learning Introduction.
-
Logic of Gradient Descent.
-
Regression Comparison: Multiple vs. Simple.
-
Estimate variability analysis.
-
Fake variables.
-
Logistic and multiple regressions: Similarities and Disparities.
-
reducing complexity of models.
-
Regression with steps.
Unit3:
Analysis of Discrimination
-
Enhanced Profiling.
-
Discriminant Function for Two Groups.
-
Assignment of Cases.
-
Model Assessment.
-
Functions of classification.
-
Squared Mahalanobis Distances.
-
probabilistic approach.
-
Reduction of the Model.
-
Common Discriminant Analysis.
Unit4:
Decision Trees
-
How do decision trees work?
-
Borland Trees.
-
Decision Tree's Qualities
-
prudence guidelines.
-
Classification Tree (CART)
-
Regression Tree, CART
-
Tree CHAID.
-
Unexpected Forest Tree.
Unit5:
Bayesian, neural networks, nearest neighbor, and deep learning
-
Conditions for probabilities.
-
Probabilistic forecasting.
-
Distance between neighbors.
-
K-nearest neighbors' distances from you.
-
Weights in a Neural Network model.
-
Hidden layers play a role.
-
Advantages and disadvantages of neural networks.
-
Deep Learning.
-
Introduction to Big Data.